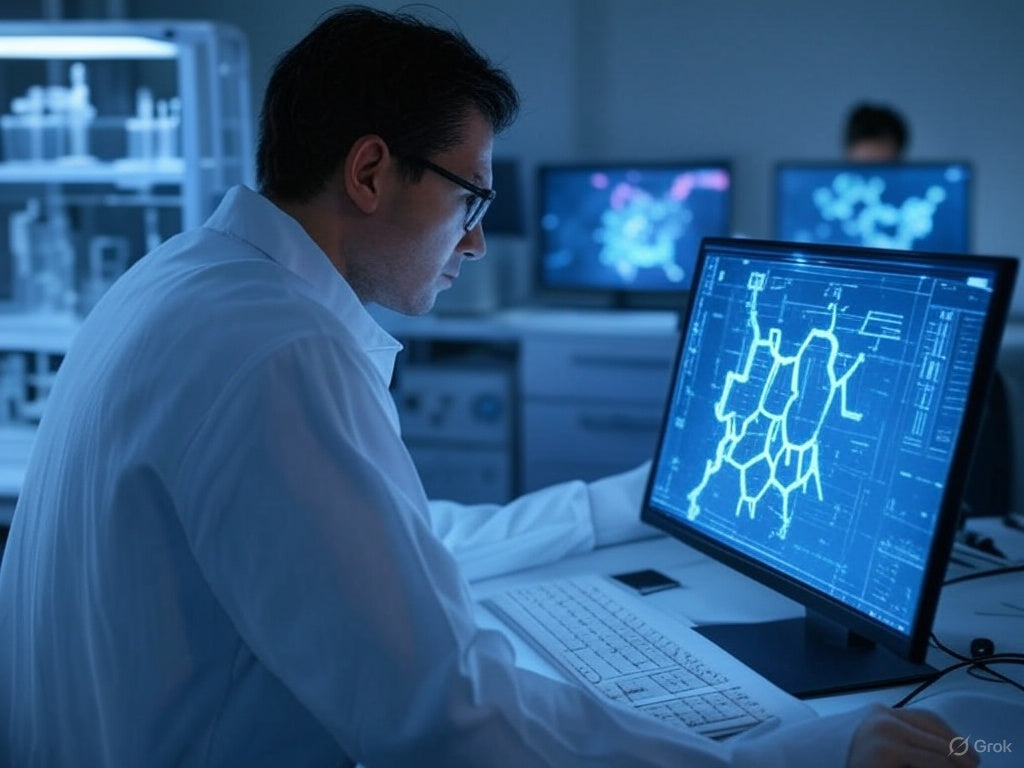
Revolutionizing Drug Discovery: How AI is Powering Antimicrobial Peptide Innovation at Bio Peptide Technologies
Introduction
Antimicrobial peptides (AMPs) are short chains of amino acids that show promise in fighting bacteria, especially those resistant to traditional antibiotics. With antibiotic resistance becoming a global health crisis, finding new ways to discover these peptides is crucial. Artificial intelligence (AI) and machine learning (ML) are revolutionizing this process by analyzing vast amounts of data to identify and design new AMPs faster and more efficiently. This blog, brought to you by Bio Peptide Technologies, explores how AI is changing the game in peptide discovery, highlighting its potential to address antibiotic resistance and the market opportunities it presents.
How AI is Transforming Peptide Discovery
AI is making waves in peptide discovery by using advanced computational methods to tackle the challenges of traditional approaches, which can be slow and costly. There are two main ways AI is being used:
-
Predicting Peptide Properties (Discrimination): AI models can predict important traits of peptides, like how well they kill bacteria, their toxicity to human cells, and their stability. This helps researchers focus on the most promising candidates without wasting time on less effective ones.
-
Designing New Peptides (Generation): More excitingly, AI can create entirely new peptide sequences. Using techniques like deep learning, it can design peptides from scratch (de novo) or modify existing ones to improve their properties. Methods like discriminator-guided filtering and latent space sampling ensure these new peptides have the desired characteristics, such as high efficacy and low toxicity.
Recent studies, such as a review in Current Opinion in Structural Biology (Artificial intelligence-driven antimicrobial peptide discovery), show significant progress in these areas, highlighting AI's role in speeding up the discovery process.
Real-World Example: AI in Action
A study published in European Journal of Medicinal Chemistry demonstrates AI's practical impact. Researchers used deep learning to screen 30,000 random peptides and identified twelve potential AMPs. Three of these, named P2, P11, and P12, were tested in labs and showed strong antibacterial activity with low toxicity to human cells. Notably, P12 was effective in a mouse model of Staphylococcus aureus wound infection, with no significant harm to organs even at high doses (400 mg/kg). This shows AI can not only find new AMPs but also validate them in real-world scenarios, offering hope for new treatments (Discovery of AMPs from random peptides via deep learning-based model and biological activity validation).
Addressing Challenges with AI
Despite their potential, AMPs face hurdles like toxicity, poor stability, and high production costs, which have slowed their use in clinics. AI is stepping in to solve these problems:
-
Toxicity: AI can predict which peptides are safe for humans, reducing the risk of harmful side effects.
-
Stability: Machine learning can forecast how long peptides will last in the body, crucial for effective treatments.
-
Cost and Time: By optimizing designs, AI cuts down on trial-and-error, lowering the cost and time needed for development.
-
Selectivity and Penetration: AI helps ensure peptides target only bacteria and can enter cells effectively, improving their performance.
Studies like those in Nature Reviews Bioengineering (Machine learning for antimicrobial peptide identification and design) highlight how AI addresses these issues, making AMPs more viable for clinical use.
Market Potential and Future Trends
The peptide therapeutics market is booming, with an estimated size of around USD 50 billion in 2025, expected to grow at a rate of 5-10% annually in the coming years, driven by demand for treatments for chronic diseases like cancer and infections. AI is set to boost this growth by speeding up drug discovery, potentially reducing costs and bringing new AMPs to market faster. For Bio Peptide Technologies, leveraging AI could position the company as a leader in developing innovative therapies, especially for antibiotic-resistant infections.
Future trends include advancements in generative AI, like diffusion models, and combining AI with technologies like nanotechnology for better drug delivery. This could lead to personalized medicine, tailoring treatments to individual patients, further expanding the market.
Survey Note: Detailed Analysis of AI-Driven Peptide Discovery for Blogging in 2025
This section provides a comprehensive analysis of AI-driven antimicrobial peptide (AMP) discovery, suitable for a blog targeting diverse audiences, including scientists, healthcare professionals, and general readers. The focus is on identifying areas with significant growth, innovation, and public interest, based on recent literature and market insights as of April 19, 2025. The content is designed to be in-depth, SEO-optimized, and aligned with the interests of Bio Peptide Technologies, a company at the forefront of peptide research and development.
Background on Peptide Discovery and Antibiotic Resistance
Peptides, defined as short chains of amino acids (typically 10–100 amino acids), are pivotal in modern medicine due to their versatility in drug development, particularly for antimicrobial applications. AMPs are characterized by a positive charge and high hydrophobicity, preferentially targeting microbial membranes, though some can be toxic to mammalian cells. Their properties, including activity, toxicity, stability, and synergy, are critical for clinical potential. The global health crisis of antibiotic resistance, with infections killing 1.27 million people annually as noted in a recent Cell article (Discovery of antimicrobial peptides in the global microbiome with machine learning), underscores the urgent need for novel antibiotics. Traditional peptide discovery methods are slow and costly, often taking over a decade and billions in investment, making AI a transformative tool.
Methodology for Identifying Trends
The analysis draws on recent publications from ScienceDirect, Nature Reviews, PubMed, and other sources, focusing on AI methodologies, case studies, and market projections. Key areas include discrimination and generation approaches, experimental validations, and market impact, with an emphasis on AMPs due to their relevance to antibiotic resistance. The trends are selected for their potential to engage readers, considering both scientific depth and public health relevance, with SEO optimization in mind using keywords like "AI-driven peptide discovery," "antimicrobial peptides," and "drug discovery."
Detailed Trending Topics for AI-Driven AMP Discovery
Below, we detail the key aspects of AI-driven AMP discovery, organized by theme, with supporting evidence and potential blog angles. Each topic is backed by recent research, and tables summarize key data for clarity.
1. AI Mechanisms in Peptide Discovery
AI is revolutionizing AMP discovery through two main categories: discrimination and generation, as outlined in a review in Current Opinion in Structural Biology (Artificial intelligence-driven antimicrobial peptide discovery).
-
Discrimination Approaches: These involve ML models predicting peptide properties such as activity, toxicity, stability, and synergy. For example, discriminators aid in identifying promising candidates by analyzing datasets, reducing the need for extensive experimental screening.
-
Generation Approaches: These use generative AI to create novel AMPs, either de novo or as analogs. Methods include discriminator-guided filtering, positive-only learning, latent space sampling, conditional generation, and optimized generation, enabling controlled design with desired properties.
Table 1: AI Methods in AMP Discovery - Key Categories
Category
|
Description
|
Example Methods
|
---|---|---|
Discrimination
|
Predicts peptide properties like activity and toxicity
|
ML models for property prediction
|
Generation (Unconstrained)
|
Creates new peptides de novo, learning from data distribution
|
Deep generative models, latent space sampling
|
Generation (Analog)
|
Modifies existing peptides to improve properties
|
Conditional generation, optimized generation
|
Recent focus, as per the review, has been on generative modeling, with detailed characterization of AMP properties and model representations, offering exciting directions for blog content on AI's creative potential.
2. Case Studies and Experimental Validation
A specific study, "Discovery of AMPs from random peptides via deep learning-based model and biological activity validation," published in European Journal of Medicinal Chemistry (Discovery of AMPs from random peptides via deep learning-based model and biological activity validation), used deep learning to screen 30,000 random peptides, identifying twelve AMPs. Three candidates, P2, P11, and P12, were validated experimentally:
-
P2, P11, and P12 exhibited broad-spectrum antibacterial activity and low hemolytic toxicity.
-
P12 showed significant efficacy in a mouse model of Staphylococcus aureus wound infection, with low toxicity to major organs at 400 mg/kg.
This case study is ideal for blogs, illustrating AI's practical impact and offering a narrative of success in real-world applications.
Table 2: Case Study - AI-Discovered AMPs and Validation
Peptide
|
Antibacterial Activity
|
Hemolytic Toxicity
|
Mouse Model Efficacy
|
Organ Toxicity at 400 mg/kg
|
---|---|---|---|---|
P2
|
High
|
Low
|
Not tested
|
Not tested
|
P11
|
High
|
Low
|
Not tested
|
Not tested
|
P12
|
High
|
Low
|
Effective (S. aureus)
|
Low
|
3. Challenges Addressed by AI
AMPs face significant hurdles in clinical translation, as noted in Nature Reviews Bioengineering (Machine learning for antimicrobial peptide identification and design), including toxicity, poor stability, limited cellular penetration, high cost, lack of selectivity, undesirable physicochemical properties, nonspecific mechanisms, and industrial waste generation. AI addresses these as follows:
-
Toxicity: ML predicts non-toxic peptides, with studies like Plisson et al. (2020) using ML to find non-hemolytic AMPs (Machine learning-guided discovery of non-hemolytic antimicrobial peptides).
-
Stability: ML predicts gastrointestinal stability, as in Wang et al. (2023) (Machine learning predicts peptide stability in the gastrointestinal tract).
-
Cellular Penetration: Deep learning identifies cell-penetrating peptides, per Fu et al. (2020) (Deep learning predicts cell-penetrating peptides and their uptake efficiency).
-
Cost Reduction: AI optimizes design, reducing trial-and-error, a key point in the review.
-
Selectivity: ML improves targeting, as in Robles-Loaiza et al. (2022) (Machine learning for the design of non-toxic antimicrobial peptides).
-
Mechanism Prediction: AI predicts protein-peptide interactions, per Lei et al. (2021) (Deep learning for predicting peptide-protein binding affinity).
-
Waste Reduction: ML optimizes synthesis, addressing sustainability, as in Ferrazzano et al. (2022) (Sustainability in peptide chemistry, current synthesis, and purification technologies, future challenges).
These challenges and AI solutions offer rich blog content, emphasizing AI's role in overcoming barriers.
4. Market Impact and Economic Context
The peptide therapeutics market is experiencing robust growth, with varying projections for 2025. Sources like Precedence Research estimate USD 52.59 billion in 2025, growing to USD 83.75 billion by 2034 at a CAGR of 5.31% (Peptide Therapeutics Market Size Expected to Reach USD 83.75 Bn by 2034), while Mordor Intelligence projects USD 43.36 billion in 2025, reaching USD 65.20 billion by 2030 at a CAGR of 7.06% (Peptide Therapeutics Market Size Analysis | Industry Report on Share & Trends). For this blog, we use an approximate figure of USD 50 billion for 2025, reflecting the average trend.
AI's role in reducing discovery time and costs is expected to boost market growth, making peptide therapeutics more accessible. The rising prevalence of chronic diseases and antibiotic resistance drives demand, with AMPs playing a key role. For Bio Peptide Technologies, this market expansion offers opportunities to lead in AI-driven innovations.
5. Future Trends and Opportunities
Future trends include advancements in generative AI, such as diffusion models and transformer-based architectures, which enhance peptide design precision. As implied in recent reviews, the integration with nanotechnology for targeted delivery could revolutionize administration. Personalized medicine, tailoring AMPs to individual patients, is another frontier, aligning with the growing interest in precision healthcare. For Bio Peptide Technologies, leveraging these trends can position the company as a leader, particularly in addressing antibiotic-resistant infections.
Additional Considerations
While the focus is on AMPs, other peptide applications, like oncology and metabolic disorders, also benefit from AI. However, AMPs are prioritized due to the urgency of antibiotic resistance. Ethical considerations, such as data privacy in AI models and sustainability in peptide synthesis, could be blog topics for broader engagement. The integration of AI also raises discussions on accessibility, ensuring these advancements reach low-income regions and align with global health equity goals.
Conclusion
For a blog in 2025, focusing on AI-driven AMP discovery, including mechanisms, case studies, challenges addressed, market potential, and future trends, will likely attract a wide audience. These topics combine scientific innovation with practical health applications, ensuring relevance and engagement. By leveraging recent research and market trends, the blog can position Bio Peptide Technologies as a go-to resource for peptide-related insights, emphasizing their role in pioneering life-saving therapies.
Key Citations
Leave a comment